Unlocking Business Insights: The Importance of Labeled Image Datasets
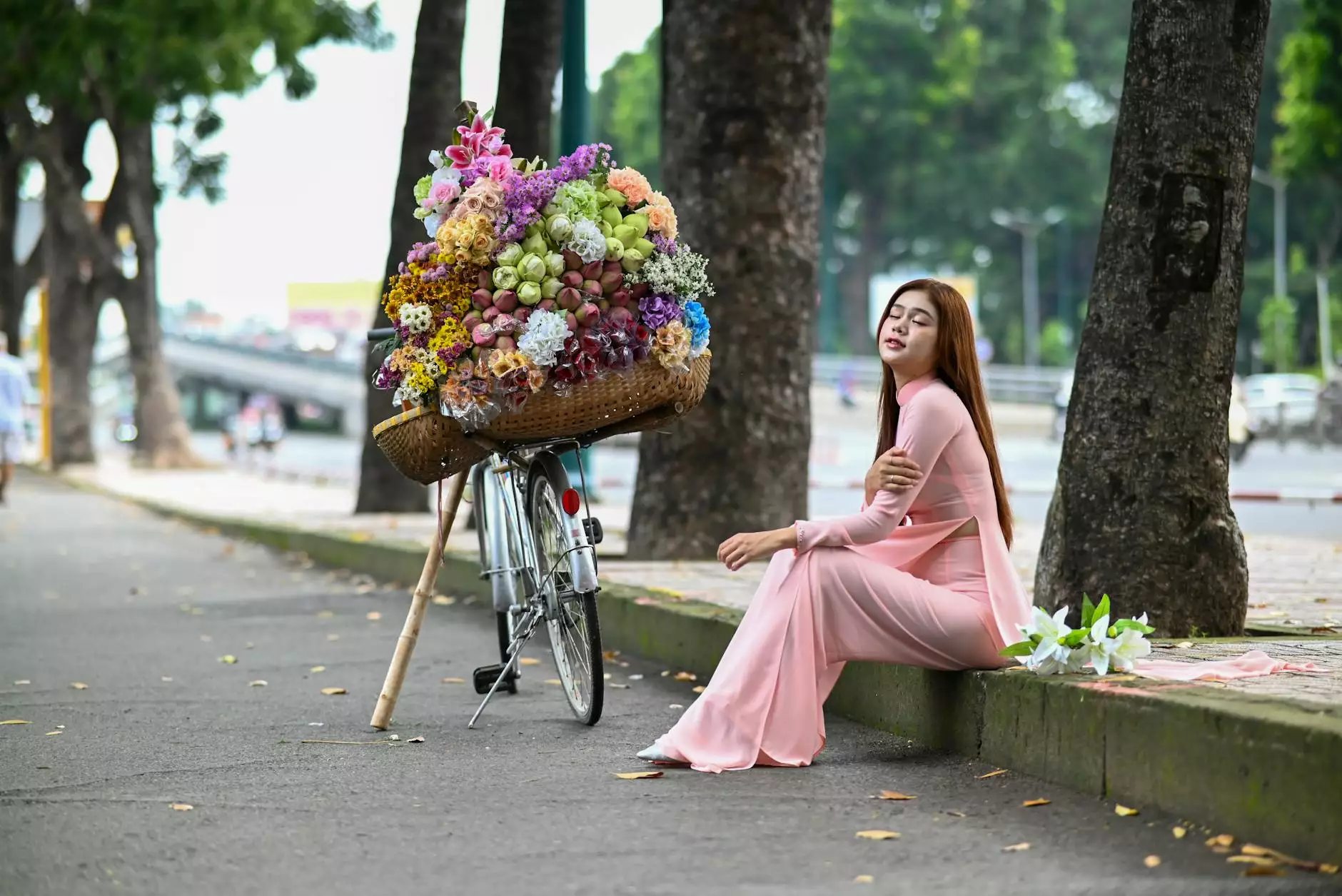
In today's rapidly evolving business landscape, the demand for precise data has never been greater. Companies are increasingly relying on data-driven decisions to guide their strategies and improve operational efficiency. A crucial component of this data revolution is the utilization of labeled image datasets, which serve as the backbone for training machine learning models and enhancing artificial intelligence applications. This article explores the significance of labeled image datasets and how businesses can leverage platforms like keylabs.ai to efficiently process and annotate data through their advanced data annotation tools.
What are Labeled Image Datasets?
Navigating the complexities of artificial intelligence (AI) and machine learning (ML) requires a solid foundation of data. At the core of this data are labeled image datasets, which consist of images annotated with specific tags or labels indicating what objects are present, where they are located, and their classifications. These datasets are essential for teaching AI models to recognize patterns, objects, and contexts within visual data.
For example, a labeled image dataset for a self-driving car might include thousands of images of road scenes, annotated with labels such as "pedestrian," "traffic light," "crosswalk," and others. Through this comprehensive labeling, the AI can learn to navigate complex driving environments and make decisions based on the visual information it processes.
The Role of Data Annotation in Creating Labeled Image Datasets
Data annotation is the process of marking up raw data to provide context and meaning. This is where platforms like keylabs.ai excel, offering sophisticated tools for businesses to create accurate and rich labeled image datasets. The data annotation process typically includes:
- Image Segmentation: Dividing an image into multiple segments to simplify analysis.
- Object Detection: Identifying and labeling objects within the images.
- Bounding Boxes: Drawing rectangles around objects to define their position.
- Semantic Segmentation: Annotating pixel by pixel to classify elements in an image.
- Image Classification: Assigning a single label to an entire image based on its content.
Each of these techniques serves a specific purpose, and the choice of method depends on the application at hand. With the rise of deep learning, the need for comprehensive and well-annotated datasets has skyrocketed.
Benefits of Using Labeled Image Datasets in Business
The use of labeled image datasets offers several advantages for businesses across different sectors. Here are some essential benefits:
1. Enhanced Accuracy in AI Models
AI and ML models trained on quality labeled datasets exhibit significantly higher accuracy rates. Accurate identification of features and objects enhances the performance of algorithms, leading to better predictive analytics and decision-making capabilities.
2. Increased Efficiency in Operations
Incorporating labeled image datasets enables businesses to automate many processes. For instance, in retail, image recognition can streamline inventory management, allowing for quicker restocking and efficient supply chain processes.
3. Customization and Personalization
Businesses can analyze consumer behavior more comprehensively using labeled image datasets. By understanding how customers interact with visual content, companies can tailor their offerings, drive engagement, and improve conversion rates.
4. Competitive Advantage
Organizations that invest in high-quality labeled image datasets position themselves ahead of competitors. The ability to leverage cutting-edge AI technologies powered by accurate data can be a decisive factor in a market filled with competition.
5. Scalability and Flexibility
When businesses utilize robust data annotation platforms like keylabs.ai, they can scale their operations seamlessly. Whether starting small or managing vast datasets, these tools offer the flexibility needed to accommodate. Along with great scalability comes the ability for real-time updates and improvements to datasets.
Challenges in Creating Labeled Image Datasets
Despite the numerous benefits, the process of creating labeled image datasets is fraught with challenges. Some common pain points include:
- Time Consumption: Manually annotating large datasets can be extremely time-consuming and labor-intensive.
- Quality Assurance: Ensuring the accuracy and consistency of annotations is crucial; errors can lead to incorrect model training.
- Resource Allocation: Businesses may outsource data annotation, which can lead to delays and additional costs if not managed properly.
- Rapid Changes in Technology: Keeping pace with the evolving landscape of AI and ML technologies can complicate data requirements.
How to Overcome Challenges with Keylabs.ai
To navigate the challenges of data annotation effectively, businesses can utilize advanced platforms like keylabs.ai. Here's how:
1. Automation Tools
With automation tools, keylabs.ai significantly reduces the time and effort required for data annotation. AI-assisted annotation can accelerate the process while maintaining high accuracy and quality.
2. Quality Control Measures
Quality assurance features, including multi-level review processes, ensure that the labeled image datasets meet the highest standards. Annotations can be verified and edited to mitigate errors, ensuring more reliable datasets.
3. Scalability Solutions
keylabs.ai provides flexible solutions that allow businesses to scale their annotation projects based on their needs. Whether you require a few images annotated or a comprehensive dataset, they can cater to your requirements.
4. Expert Annotation Workforce
Beneath the platform is a team of experienced annotators who specialize in various industries. This expertise ensures that your datasets are not only accurately labeled but contextually relevant, tailored to your business needs.
5. Continuous Learning and Support
As technology evolves, keylabs.ai stays updated with the latest trends in data annotation. Continuous learning opportunities are provided to businesses to ensure their data practices remain cutting-edge and effective.
Real-World Applications of Labeled Image Datasets
The versatility of labeled image datasets spans across various sectors. Let’s dive into some compelling real-world applications:
1. Healthcare
In the medical field, labeled image datasets are critical for training algorithms that can assist in diagnosing diseases from medical imaging, such as MRIs and X-rays. These datasets facilitate early detection of conditions like cancer, resulting in timely treatment and improved patient outcomes.
2. Automotive
The automotive industry relies heavily on labeled image datasets for technologies like autonomous driving. Through the analysis of labeled images, vehicles can learn to interpret road signs, detect pedestrians, and navigate complex traffic scenarios.
3. E-commerce
E-commerce platforms benefit from labeled image datasets for visual search engines. By analyzing product images, consumers can search by image and find similar products, improving user experience and boosting sales.
4. Agriculture
In agriculture, labeled image datasets are being used for crop monitoring and disease detection. Drones capture aerial images of crops, which are then analyzed to determine the health of the plants, enabling farmers to make informed decisions on resource allocation.
5. Security and Surveillance
Surveillance systems deploy labeled image datasets to power facial recognition technology, improving security measures. Enhanced capabilities in identifying individuals or detecting unusual activities bolster security protocols across various sectors.
Conclusion
The relevance of labeled image datasets in modern business cannot be overstated. With the ability to enhance the accuracy of AI models, improve operational efficiency, and provide competitive advantages, investing in high-quality datasets is essential for any forward-thinking organization. Platforms like keylabs.ai offer comprehensive solutions for data annotation, ensuring businesses are well-equipped to harness the power of data in their strategic endeavors. As the future unfolds, companies that prioritize accurate and well-annotated datasets will undoubtedly lead the charge in innovation and growth.